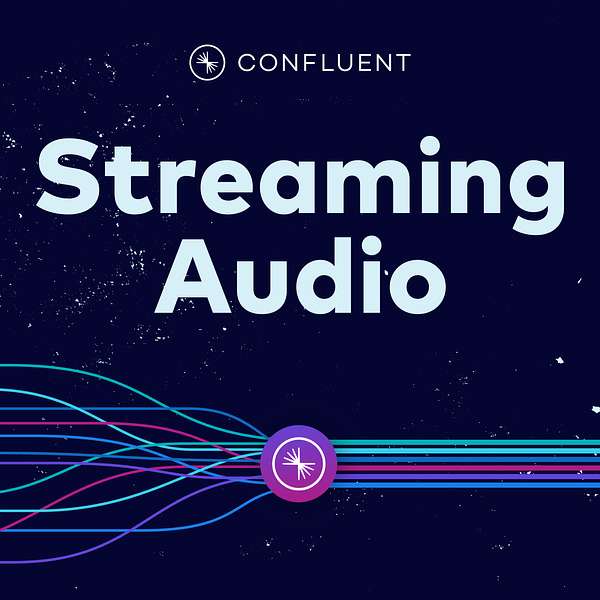
Streaming Audio: Apache Kafka® & Real-Time Data
Streaming Audio features all things Apache Kafka®, Confluent, real-time data, and the cloud. We cover frequently asked questions, best practices, and use cases from the Kafka community—from Kafka connectors and distributed systems, to data mesh, data integration, modern data architectures, and data mesh built with Confluent and cloud Kafka as a service. Join our hosts as they stream through a series of interviews, stories, and use cases with guests from the data streaming industry. Apache®️, Apache Kafka, Kafka, and the Kafka logo are either registered trademarks or trademarks of the Apache Software Foundation in the United States and/or other countries. No endorsement by The Apache Software Foundation is implied by the use of these marks.
Streaming Audio: Apache Kafka® & Real-Time Data
What is Data Democratization and Why is it Important?
Data democratization allows everyone in an organization to have access to the data they need, and the necessary tools needed to use this data effectively. In short, data democratization enables better business decisions.
In this episode, Rama Ryali, a Senior IT and Data Executive, chats with Kris Jenkins about the importance of data democratization in modern systems.
Rama explains that tech has unprecedented control over data and ignores basic business needs. Tech’s influence has largely gone unchecked and has led to a disconnect that often forces businesses to hire outside vendors for help turning their data into information they can use. In his role at RightData, Rama worked closely with Marketing, Sales, Customers, and Leadership to develop a no-code unified data platform that is accessible to everyone and fosters data democratization.
So what is data democracy anyway? Rama explains that data democratization is the process of making data more accessible and open to a wider audience in a unified, no-code UI. It involves making sure that data is available to people who need it, regardless of their technical expertise or background. This enables businesses to make data-driven decisions faster and reduces the costs associated with acquiring, processing, and storing information. In addition, by allowing more people access to data, organizations can better collaborate and access tools that allow them to gain valuable insights into their operations and gain a competitive edge in the marketplace.
In a perfect world, complicated tools supported by SQL, Excel, etc., with static views of data, will be replaced by a UI that anyone can use to analyze real-time streaming data. Kris coined a phase, “data socialization,” which describes the way that these types of tools can enable human connections across all areas of the organization, not just tech.
Rama acknowledges that Excel, SQL, and other dev-heavy platforms will never go away, but the future of data democracy will allow businesses to unlock the maximum value of data through an iterative, democratic process where people talk about what the data is, what matters to other people, and how to transmit it in a way that makes sense.
EPISODE LINKS
- RightData LinkedIn
- The 5 W’s of Metadata by Rama Ryali
- Real-Time Machine Learning and Smarter AI with Data Streaming
- Watch the video version of this podcast
- Kris Jenkins’ Twitter
- Streaming Audio Playlist
- Join the Confluent Community
- Learn more with Kafka tutorials, resources, and guides at Confluent Developer
- Live demo: Intro to Event-Driven Microservices with Confluent
- Use PODCAST100 to get an additional $100 of free Confluent Cloud usage (details)
Kris Jenkins (00:00):
Hello, you are listening to Streaming Audio. And in today's podcast, I think it's time to put another buzzword on trial. We've probed data mesh a few times on this podcast. We've gone looking for the servers in serverless. Well, this week, we are going to take a look at the rising buzzword, data democracy. What's data democracy? It sounds like data where everybody gets a vote, and I guess in a way it kind of is that. But it's also a bit like data mesh. It's partly data. It's partly about people and process. It's also partly about tooling and access to data.
Kris Jenkins (00:39):
Well, joining me to try and untangle this web and see if we can make sense of it and find the substance in a data mesh is Rama Ryali, who's the vice president of product and strategy at a company called RightData, and I guess you could say their strategy depends a lot on putting the right data into the right hands around an organization so he ought to be able to make the case for us. As ever, this podcast is brought to you by Confluent Developer. More about that at the end. But for now, I'm your host, Kris Jenkins. This is Streaming Audio. Let's get into it. Joining me today is Rama Ryali. Rama, how are you doing?
Rama Ryali (01:23):
I'm doing good, Kris. How are you today?
Kris Jenkins (01:25):
I'm very well. We're enjoying a bit of sunshine, rare sunshine in the British autumn here, which is good. So I've brought you in. You are... Let me get this right. You're the vice president of product evangelization and strategy for RightData.
Rama Ryali (01:40):
That is right.
Kris Jenkins (01:41):
I haven't brought you onto evangelize the product, but I have brought you on to evangelize an idea because there's an idea I know you've been thinking and writing a lot about, and it's one of those things which could be a buzzword or could have some meat to it so you get to convince me that it's got some meat to it. Data democratization. What does that actually mean? Why should we care?
Rama Ryali (02:04):
I think you could look at it from a... It's more of a perspective. People say it's still a buzz, but to me, it is where things happen. If you look at from the point of the data consumption because that's all about data, you could have petabytes of data, but if the data is inaccessible, the data isn't in a form where the business can gain value from it, it's no point. It's like you having a lot of money but you don't know how to make wealth. Same thing if you have data. If you don't know how to gain knowledge out of the data, it's useless. And that's where the whole notion of data democratization comes in. You could tackle data democracy from multiple points of views, either providing the right access to the right people at the right time or how do you curate information so that right information to the right people at the right time is happening just when it's necessary. Excuse me.
Kris Jenkins (03:05):
But I don't know. So I can see that you want to get data into everybody's hands in an organization in the right form. I just don't know that we can ever successfully make that truly democratic. We've been trying for years to get the tools right to put it in everyone's hands and it always falls down to a level of expertise of some kind. So what's the way forward?
Rama Ryali (03:33):
I think the word expertise, yes, you need to understand what need to be put into focus. So you need to have some knowledge into governance. I know governance is such a big buzzword. I can safely say not many people really wants to hear the word governance, especially when you go in the executive circles. They're like, okay, let's talk about the more tangibles. What are you talking when you say governance? What makes sense to me or the organization? So I think to your point, yes, there are specific areas of data democracy that needs some technical savviness.
Rama Ryali (04:13):
Example, the tools and the enablement of the tools is technical in some way, whether it's capturing a lineage, whether you want to do change management, whether you want to do data modeling or solution architecture and then metadata... There's certain facets, or data quality to me is so big you can't overlook it. I think to me, even me as an engineer, as an architect, though I hold those close to my chest meaning those are more high priority to me, I've never seen an organization that has succeeded from its data consumption initiatives without having a focus to data quality. So data democracy, yes, it's loaded. But given how the landscape is coming together from a technology evolution standpoint, it is not as complicated as it used to be.
Kris Jenkins (05:10):
That kind of makes me think almost... I'm forming a diagram in my head that's almost tiered where data quality is on the way in, data modeling is at the very heart of it and data democracy is more about the consumption and output. Do you think that's fair?
Rama Ryali (05:29):
In a way because for data democracy to succeed from a data literacy standpoint, yes, consumption holds the top in the list of the things as priority for you. Why would you want to build a product... And I'm kind of jumping into or leading us into the next in, what's coming up from the whole notion of being a data product. Why would you want to build a data asset as a product if there is no consumption? Why would people care to know about the data if it's not something that the business needs for it to grow? So from that point of view, you certainly want to put higher emphasis to the data that is more value add from a business success standpoint. So data quality doesn't have to be an emphasis on anything, everything as a data. You probably have, out of the few hundred data that you accumulate in the enterprise, it could be a handful that are absolutely no brainer that you have to put the emphasis into architecture, data quality, data modeling, change management, more emphasis.
Kris Jenkins (06:44):
I'm thinking like sales figures. That's one just about everyone can agree on.
Rama Ryali (06:48):
Absolutely. I was going to go with that example myself. Yes.
Kris Jenkins (06:53):
But then you get into the problem of that kind of slightly more peripheral data where you need a developer who's an expert to surface it, but you need a business person who's an expert to know which thing to surface. And sometimes it feels like there's no hope of the two meeting in the middle.
Rama Ryali (07:14):
That's a good point also because yes, the viewpoint of a DBA is very different from a viewpoint of a business analyst. For a DBA, it's natural. Oh, I know when my table got created. I know who has access to it. I know what permissions I need to manage, what profiles I need to create. That's common sense. I know how to access data. I know how I could reverse read back into the data through the ETL tools. There's many different ways to do it. But from a business user standpoint, I don't care about all that. I have a need to reach into and read my sales information, taking that same example, over the last three years. Because if I'm looking at my revenue at a store year on year, month on month, my needs as a business is different from how you as a data engineer or a database administrator or a data architect would look at the information.
Rama Ryali (08:10):
I don't care about the billions of records in the table. All I need is those few thousand summarized views of information. And from a business standpoint, I need to be in a position to self-service myself. Meaning when I'm looking at the sales summary table, is there enough information on these 50 columns that get read through a UI interface, AKA Power BI, a Tableau, a Qlik or any one of those legacy or more current legacy, in the sense, business objects. They still exist. The Obs. They still exist, Cognos and things. End of the day, it's the same question from the business. Beautiful billion record table. I have no clue how to read even a record on it because I don't care about how complicated the technology is. Give me the simple soups and nuts, not in that level, but more from a dictionary standpoint where I can make some sense because these people in the technology are super busy so you can't expect their business to wait for months to answer a simple question.
Kris Jenkins (09:17):
Yeah, it's true. Okay, so let me think about this historically. So we had this dream back in the '70s that we would invent this thing called SQL, and everyone in the business would just select the things I'm interested in from sales table where region is the United States. And that was sold as the dream that everyone would be able to access their data that way in different ways. Never really worked out. I've met a few people in sales and a few handful of people in sales and marketing that can write SQL. The rest look at you like you're speaking Latin, which you probably are in a way. An ancient language that's never going to be alive to them. That leads me into thinking about tooling and I'm not sure I believe this, but I'm going to challenge you with it. We would be better off if we just managed to dump every possible data source into Excel. Excel is the only tool that's actually succeeded in making data democratically available to nearly everyone. Is that fair? Is that crazy?
Rama Ryali (10:23):
No, I think it still happens. I think one of the challenges for any data solution or data value extraction is the simplicity of access to information. And you're right, Excel is still that tool that makes it easy for most people because Excel has penetrated so deep into the business, into the technology spaces. People think it's easy. Yes, to a point, but then you need to remember. Excel can help with some simple data massaging, data cleaning, data enrichment to a point, but Excel has way too many limitations. One, in order to get the information into Excel, either you need to have access to a database which could be a SQL again, or you need to have someone provide you the information through an extract or something. So I think you need to pay attention to that whole notion of you're depending on someone to give you the information.
Rama Ryali (11:32):
So if this information and example of sales is changing constantly, how do you keep up with it? How do you upkeep with that. Excel though is great, but that latency, the stainless, the freshness, if you look at those dimensions from a data quality standpoint, how do you manage it? How do you explain it to executive representation that you're presenting a view of something? Take this Excel data, put it into some reporting platform in some tool. How do you justify the numbers? Because you have one number, I have one number. Because my numbers are probably five-minute delay, and your numbers are probably a week delay, whose numbers are right? From your point of view, from the context that you have, you are right on. But that is a challenge. Excel though is great, SQL though is... It's interesting. I'll come back to SQL in a minute. But that's just the way I see. The challenge is only becoming bigger because the resistance on people saying I could do all this in Excel, I would challenge that to say, "But how do you guarantee the trustworthiness of information?"
Kris Jenkins (12:41):
Yeah, yeah. I see that. I still think Excel has a great discoverability property of information. So is the solution then that we should build a real time read only streaming version of Excel?
Rama Ryali (12:58):
Excel is a tool. Let's not forget that. Excel is based on the memory on your machine. So these days, I know memory is cheap. You don't have to look for. Computers come very fast. But still, Excel, the way it's built, whether it's 64... They say everything is 64 bit. You still run into that roadblock. I know that number is pretty ginormous. But tell me, one, let's say you have an Excel with a 100,000 rows. How many records you think as a typical analyst you would really glean at to make some sense of the information? Probably Excel does the typical algorithm in Excel. It looks at the first 200 rows in the spreadsheet and it starts to make some assumptions.
Rama Ryali (13:46):
And you know what's wrong with that? The first 200 might be perfect. This is the same challenge a data scientist would run into because when they do the profile of information, they look at the first 200 and they make a lot of assumptions and the assumptions are normally not almost right every time because data changes, because what you think is a number probably came in as a number for 200 records. But because the Excel is making assumptions saying I'm going to treat you as a number, and all of a sudden you get an alpha or an alpha going in as a number, which is a bigger problem.
Rama Ryali (14:20):
So you have those challenges. Excel though is great, but Excel is one tool. Excel is limited by how much computing you have in your machine. There are challenges. You could do anything in Excel. I still have people who built phenomenal dashboards coding in Excel. I've seen people who lived all their life saying, "I don't want to do anything but in Excel." And these are engineers I'm talking about. So I think that's a difficult thing because Excel, to your point, is a phenomenal BI tool and that probably leads into the Power BIs and everything why they came out saying, "Oh my God, this is better than that. Let's do something and extend it into it." So I think the evolution has made it simple. The Tableaus, the Power BIs, the Qliks, they're much simpler to use and there is no question about it. Excel can do it, but Excel has its own limitations.
Kris Jenkins (15:14):
Yeah, true. Okay, let me branch into another thing I know you've been thinking about. If one of the problems with Excel is it gives us a limited snapshot view over things and our other problem is we've got to discover which bits of the data we should be interested in, is the next real solution to data democratization machine learning where it can handle a sample of data and predictions about the value of it, where it can handle discovering which columns are more interesting than others? Should we be running everything through unsupervised learning in the hope that it tells us which questions we should be focusing on?
Rama Ryali (15:58):
Good question. But let me-
Kris Jenkins (15:59):
Sorry, [inaudible 00:16:00] here.
Rama Ryali (16:01):
No, no, I think this is good because I'll tell you why. Machine learning is exciting, but when you look at the stats, machine learning is still niche. If you think SQL is niche, machine learning is even more niche. You really need to understand your ABCs on mathematics, especially these Bayesian theorems, everything. How you do the clustering or classifications? They're not as simple. You need to have understanding of pythons. You need to understand some level of Spark or Scarlett, some level of coding though I call it the end generation, the fifth generation of coding, unlike the C++ days when I used to be a C++ developer, even coming into .net was like, oh my God. And then going into Java was, oh my god, even better. So these are becoming easy, but easy is a perspective.
Rama Ryali (16:56):
So not everyone can thrive to be a data scientist, but one thing I absolutely see a challenge. If your data is crappy, garbage in, garbage out, no matter how great your data science algorithms are... And you probably have heard enough stories or audience probably have heard enough stories where organizations say AI can solve everything for us. It's never realized the dream. For one, the biggest challenge I was going to go to is when you look at a typical data scientist, this is a stat. I can't remember if it is Gartner, whether it's one of them, but it's a known fact. 85% of the time a data scientist invest is data preparation, data cleansing, data [inaudible 00:17:42]. 85%. They typically barely spend any time to build a true model that someone can gain value or glean any interest into. That's the challenge. Yes, AI can solve everything probably, but this challenge of data quality, this challenge of lack of knowledge into how I self understand the data, this challenge of data science being a niche technology suite of products or knowledge, it's still going to be a challenge.
Kris Jenkins (18:16):
Okay. So yeah, I think that makes me temporarily more depressed because our hope of getting data democratization, you're saying we are tackling the 15% of the problem. We've still got the 85% of the data isn't worth being democratically available yet. So is there some way? Tell me you've got some answers, some solutions forward to improving the state.
Rama Ryali (18:41):
If you wouldn't mind, let me read through one of my white papers. What are we talking from a data democracy standpoint? I think one is the sense of people... I'm glad for a point. Though we don't call it data governance, the metadata management, the cataloging information is becoming such a big thing. Even you look from a data science perspective or a general business analyst perspective, the challenge is there is data in the enterprise. Data is there. The data that they need is right there, but how do we help them learn the data on their own and make some meaningful decisions quickly? So the cataloging the metadata management is necessary. I think you need to figure out data governance is no more the waterfallish data governance.
Rama Ryali (19:37):
It's just how do you get into the data governance as just in time? You don't have to spend 18 months to formalize the data governance practices. How do you look at an asset by asset point of view as a data, as a product, everything you want to get a value from? How do you define enough information on the asset whether you want to define a data model? I'm a big believer of data modeling from a functional data architecture standpoint because that's the first step where you get the requirements and you take the requirements before you go even build anything, you're putting a logical, conceptual, visual representation of the solution. So a business less savvy, even a data scientist who is very niche in one specific area would get to see it and say, okay, here is the technology team that's building a data product for me. Here is where an asset is being bought into some central location. And here is, because of the criticality of the asset, here is a data model.
Rama Ryali (20:36):
Let me look at the data model. Let me align that with the requirements that were given to solve this and is it making sense. That's a engagement point. I would say to answer the question that's the first engagement point for a non-technical business, non-IT person looking at that and saying, is this solution viable? Am I answering my questions through this data model? Is there a solution diagram that shows me how the information flow happens at a high level? To me, those are basic and fundamental depending on what kind of asset you're trying to build, especially for the assets that are high critical, high value.
Kris Jenkins (21:11):
So are you saying, in a way, we've got to have a core model that makes sense to everyone and the lineage to back it up?
Rama Ryali (21:20):
Lineage and the metadata. So the context. If we look at the sales table, what is the date on the sale? If there's a column called date, it's simple. What is it? Is it a date of putting an order or is it a date of shipment? Is it a date of purchase? Whatever I think is, that context needs to be captured and needs to be centralized into some metadata management tool. Back to the lineage. Of course, you probably might ask me the question saying you can't boil the ocean. Yes. This is where the experience and the knowledge of the technology teams comes to the forefront to say how much is enough?
Rama Ryali (22:03):
It's like everything is iterative, everything is agile, so you need to block it out saying what is possible in a release cycle which is typically six weeks to three months? So what is possible in that release cycle? Am I giving incremental value to the end consumer during each of the iterations, each of these prints? I think you need to simplify the process. It's as simple as it sounds from what I'm saying. Yes, it has its challenges, but I think this is where the common consensus as part of the overall buy-in, either from the business or the technology to come into play to say what is enough? Let's define that. And it should not take months and months to define it.
Kris Jenkins (22:48):
So that plays into the idea of agile, right?
Rama Ryali (22:51):
Absolutely.
Kris Jenkins (22:52):
But I think it also plays into... We're going to hit our bingo card of buzz terms here, but I think it's an important one. This idea of event storming. This idea of you've got to have a shared language between the technology people and the business people. So democratization is not just about everyone having a voice, but everyone talking to each other with that voice.
Rama Ryali (23:18):
It's more of, I think to me, collaboration is such a big thing in data spaces. I think the notion that we build, they come, I've never seen that being fulfilled. I've never seen that. So I think that balance need to exist. The way I always see an IT, IT is just an enabler. IT is just a steward with a good intention that we want our partners to succeed and our goal as IT is to bring you a solution that is easy to follow, not too expensive because the total cost of ownership cannot be overlooked. Data democracy. Let's say the organization has 20, 30 business units. We don't want the 20, 30 business units to go buy their own products.
Rama Ryali (24:07):
That's not going to help you either because end of the day, technology has to support the product from a oversight, from a governance, from a management. That's not easy. So we need to come to an agreement. This is part of the democracy, is what are the tools that make sense from a query standpoint, from a data management standpoint, data access standpoint, data visualization standpoint? If you are making it a self-service model, what technology you want to bring in so the business can go build their own data pipelines? How do you solve that because the challenge is there is never going to be enough people in IT to solve every problem the business has? To me, data democracy is not just about accessing the information, but how do you give the safe and secure access to the end consumers also so they can be self-sufficient. You need to balance it. That act to me is democracy.
Kris Jenkins (25:01):
So let's make this more concrete. Have you seen anyone that's done this well or instructively badly?
Rama Ryali (25:10):
I can take my own example. I won't name the name of the organization. But yes, we certainly were on the journey. This is where one of the very big organizations was making a transition from a on-prem mainframe to a cloud. It was tried many times.
Kris Jenkins (25:25):
Can you tell us the sector?
Rama Ryali (25:27):
This is in the hospitality.
Kris Jenkins (25:28):
Okay.
Rama Ryali (25:30):
I believe we were doing phenomenal things. We were certainly from an architecture standpoint. We said, "Here are the boundaries. We're going to limit ourselves and we want to make it agile. We want to keep the cost low. We want to standardize on technology." We were about 60% to 70% successful. In that same thing, we did a extrapolation just to see what the savings would be for the enterprise. So we took the math and said, let's assume we build this metadata product. We were building a centralized catalog to make sure accessibility data democracy are always on the table.
Rama Ryali (26:04):
And the math was, let's say we save 15 minutes, one five, a week on every analyst, and our math was on average, this enterprise had about 3000 analysts, whether it's technical, non-technical, business. And 52 weeks, 15 minutes roughly comes to 13 hours a year per analyst. So 15 times, we did the math, $60 an hour for analyst time. So it came around $9 million of saving just 15 minutes a week of saving. So the key is how do you identify what is that absolute necessity? That always to me has been the biggest challenge. And also recognizing tools will not solve anything or everything for you. Tools are just a mechanism to make it easy on you. Identifying the right tool is absolutely as critical as making sure you're enabling the end consumer at an equal pace. So they're also like, okay, we get it.
Kris Jenkins (27:11):
So how did you go about that? What was the 15-minute window that you looked for? How did you find it?
Rama Ryali (27:17):
We were just having these conversations with the business, the domain users, the end consumers of the data, and we started asking them the questions because we had this catalog which had data models, metadata including lineage, the business glossary, everything we were trying to bake into this. And as part of the roadshow, as part of the penetration, as part of the outreach, we would have these weekly conversations with the business stakeholders on a very frequent basis to say what is working because we were trying to standardize a stewardship model because we wanted to make sure the end consumer has full control on their own data assets like a finance, a sales, like marketing. If you are in that space, you are the steward. You control access to information. So I think we were trying to make that a standard within the enterprise. So as we were having these conversations, our questions was what is the least amount of time we can look at as an increment?
Rama Ryali (28:16):
And 15 minutes was nothing. We knew 15 minutes was nothing because we were trying to make a case to show how this is helping the enterprise. At end of the day, data democracy and data monetization... I know I'm going into another facet. They're not very different. You could gain data monetization a different way also. This efficiency that we gained showing a 15-minute saving a week by analyst, if that was $9 million, which is hardly anything for that big enterprise, but it's still money that could be reinvested into doing something different. That was a point we were trying to make. 15 minutes was nothing. We could probably be saving an hour a week or maybe three to four hours a week. We knew it, but we just took the lowest possible value and we started to show the value proposition.
Kris Jenkins (29:07):
I should double-check at this point just for the factual record, the IT project, I hope, costs less than $9 million?
Rama Ryali (29:17):
In certain way, probably you're right. In this case, the amount of investment the IT was making was a big team. We were around 60% more than that. $15 million, $16 million. So it was a good chunk of the dollar investment. Yes.
Kris Jenkins (29:33):
Okay. So you got a decent return on initial investment?
Rama Ryali (29:37):
That's what we were projecting, and I hope that's how the finance was actually recuperating that value back from the investment.
Kris Jenkins (29:46):
Okay. So that point of order aside, it sounds to me, yet again, like a large part of this is talking to different sections of the business in an ongoing way to figure out which data needs to be democratized.
Rama Ryali (30:05):
Yeah, if data asset accumulation is from the viewpoint of data consumption. Data driven to me is such a overused term. To me it's more data consumption driven, less of data data driven. Data consumption driven viewpoint, I've never seen... Actually, let me say this. I've hardly seen that gone wrong. When you look at the point of what is a consumption need and let's focus on that, it helps in one way. Actually, helps in more than one way. One is giving you the focus for the limited IT staff or the staff that builds the solutions. And the two, you're building data asset, a product or whatever so the end consumer is really using it. In most cases, as we all seen as seasoned experts in the data, is the business probably says, "Yeah, I need this data." And then probably in three to six months they're like, "No, this is not what I needed." But at least you had a consensus.
Rama Ryali (31:10):
Everything starts with the budget. The budget is where the business comes into play, the use case, the seed or the ideation and everything. Yes, that needs to happen because you need the funding from an IT standpoint. Rarely an IT team is self-funded. It's normally coming through some business initiative. So all that is adding up. Yes, probably there are times when what you think upfront makes sense three months ago, but then the business focus has shifted and no more that asset is valued. Yes, it happens on and off, but generally speak, you are not disconnected. So there is an equal share of responsibility at the end of the day. More of success, it's like how do you manage and set expectations to me is much bigger than giving a fancy solution. So in that way, you're not completely lost on the end outcomes.
Kris Jenkins (32:04):
Yeah. Okay. Do you know what this is making me think? And this is a stretch. Feel free to tell me if I'm stretching the metaphor too far. But in order to have data democratization, in order for the data to permeate where it needs to go, we need to have these iterative cycles. We need to be talking with different parts of the business. We need the tools that enable those conversations and that visibility. And I start to think with all this back and forth and social interaction on top of it, maybe a better term than data democracy would be data socialization because we have to keep the human angle in.
Rama Ryali (32:46):
I kind of like it. Maybe I'm going to start using that saying data socialization as part of data democratization or maybe data socialization is such a big, big part of this because it's all about human connections end of the day. I'm a strong believer. Agile... I'm sorry, you can cut it off. But agile is fragile. I've always believed in it. Yes. Agile is become such a basic necessity for a different reason. Agile started for a whole different purpose, but then it's evolved and it's been adopted by everyone, anyone for the right reasons. But agile can be fragile, and this is exactly where it can be fragile because those conversations as part of socialization are so essential.
Kris Jenkins (33:38):
Yeah. I look at agile development in the wild and I don't see much I recognize. I go back and read the agile manifesto and it says, "We value people more than processes." And that's going to be a perennial truth, I think.
Rama Ryali (33:54):
I think the agile definition for people, process is the team itself. But to me, the team in the data space unlike in engineering, a typical software, of course the lines are blurring between the software engineering and data engineering because the tools have evolved so much. APIs are becoming so much of the backbone for everything you're doing. The mere realtime consumption is such a new norm. I wouldn't call a norm. It's pretty much a standard these days. So with all that, agile still has its place, but in data, because data is such a interesting commodity, it needs a lot of collaboration.
Kris Jenkins (34:35):
The odd thing about data, I suppose, is it has such a long life cycle. It tends to outlast all of the players.
Rama Ryali (34:42):
Yeah. Application's an example. How many times I've seen applications that kind of sunset, but the data continues to exist?
Kris Jenkins (34:52):
Yeah.
Rama Ryali (34:53):
I've remembered... Oh my God, this is a telecom company. We wrote some solutions back in 2007. And my understanding is those solutions as interfaces don't exist, but the data is still generated. And it is 16 years since that process is still running and there are here is the only process that chunks that kind of data out for the whole enterprise.
Kris Jenkins (35:19):
I can believe it. I can easily believe that. I'm sure if you go into plenty of large banks, you'll find systems that are pumping out data from 40 years ago.
Rama Ryali (35:27):
Yeah, yeah. True. Very true. There you go. That's a great example too. Yeah.
Kris Jenkins (35:31):
So let's make this concrete one last time. Maybe you should tell me where RightData fits into this. I'll give you your chance to shine in the solution here.
Rama Ryali (35:43):
So I'll just give an introduction of RightData to begin with, and then I go into how RightData supports this. So RightData is a software company that builds trusted software that empowers end-to-end capabilities for the modern data analytics and machine learning in the Lakehouse, and we have two technologies to support it. One is a data engineering platform called Dextrus, D-E-X-T-R-U-S. And the second one is RDT, as in RightData Testing Tool. That's from a testing automation standpoint. Observability and testing standpoint. The combination of these two as RightData from Dextrus and RDT is what makes it possible if someone wants to adopt into a Data Ops methodology.
Kris Jenkins (36:37):
Data Ops, new buzz term. Define that for me.
Rama Ryali (36:40):
So DevOps is known. It's that infinite loop where you build and you manage the product using tools, technology and the teams are kind of intermixed in a way that you develop and you support it on their own. But that's DevOps to a point. But Data Ops is to the power of two, to the power of n of DevOps. The challenge with data is one, yes, you need to have a infinite loop on managing your data assets like an engineering standpoint. And then from you as or I as an end consumer of information, how do I provide the notion of innovation into the data development from the DevOps standpoint? You have data science team, you have business teams. They have a need to innovate. They need to self-service. They need to explore the data. So what the Data Ops says is you have one component of building these products on steroids and the other component that feeds into this pipeline because it's continuous integrations of data.
Rama Ryali (37:48):
As continuous integrations happen, how as an end consumer of information, how can I do my ideations, the exploration as all this happens and how this sinks into the continuous evolution of data standpoint? And how do I build platforms? You see the whole database as a service, a data as a service. How do I through the containerization or whatever the approach you're going to take on the cloud deployment of solutions... Can I stand up my own ecosystem as a developer, as an analyst? So I have my sandbox environment ready for me. So I am self servicing. All this ideation, engineering, my self discovery standpoint as a sandbox standup. Machine learning needs everything. This all put together, the ML Ops, all that in the equation, how can they continuously be iterating, evolving, maturing, to me is Data Ops.
Kris Jenkins (38:54):
Right. Okay. Yeah, I can see that. This constant ongoing cycle of discovering new things, perhaps generating new hypotheses about your data, discovering faults in the same way that we constantly maintain our servers in DevOps. Okay, that makes sense to me. And you're working on a tool suite platform to enable that?
Rama Ryali (39:21):
Yeah. So for us, the engineering platform and the data quality automation tool, if you combine these two, you would get into the Data Ops methodology adoption. Of course, there are certain things you need to do in between like metadata management practices which will not have a metadata management tool. We're not a cataloging tool. Let me say it that way. Yes, we generate metadata. We have [inaudible 00:39:48] graphs into the product in Dextrus as you build your pipelines. The RDT has means to reconcile and profile and do all of that from a testing standpoint. You could do rules, automation, everything together, but independently, they do what they do. From a DevOps standpoint, here is your Dextrus. From a data quality standpoint, here is RDT combined is what you can get the Data Ops capability.
Kris Jenkins (40:21):
Okay. So let's wrap this up perhaps by going back out into the real world. You must be going out there and talking to companies with this suite of tools. But what problems are they facing? What problems are you hearing in the field? And how does the idea of Data Ops, data democracy, how is that going to solve their problems?
Rama Ryali (40:46):
So one area where we are talking quite heavy these days is data observability. I know it's another buzzword. It's kind of hard to define. To me, data observability is if you look at the whole PDMA, the plan, deploy, monitor and act, which is your Deming's model, that's pretty standard in data quality. So data observability says how do you crunch it so you're not reacting but you're proactive on the data quality improvement? So from that standpoint, data observability is one area that we're putting a lot of emphasis on. Whether it could be augmented data quality or whether it's data quality that comes through the rules you capture into your product suite like the RDT here in the example, or from an engineering standpoint, how do you put your solutions from a simplicity point of view? You don't have to be a deeply savvy engineer using these.
Rama Ryali (41:53):
In the old days... I'm calling out a few technology partners here like the Informaticas of the days or the SSIS of the days or DataStages of the days, they were very heavy from adoption standpoint. Technology like Dextrus, they're so simple. Yes, it's still an engineering platform, but it's not as complex. It's drag and drop interface, very user friendly. Yes, you can still code in it, Spark and everything. But I think this simplicity of adoption of platforms from a data engineering standpoint to me is all enabling this process of data democratization going back to the point and, of course, looking at from a data observability when you have tools like RDT, which bring in that focus into the trust of data through data quality.
Kris Jenkins (42:42):
Right. So I'm wondering is that the solution or the symptom? Are you actually going to business and they're saying, "Our biggest problem is we can't observe our data." Or is there another symptom for which observing the data would solve?
Rama Ryali (42:59):
So the observing data is just one of the problems we are looking at. Back to your question, most of the enterprises, even technical people, data observability is a challenging statement. They won't come to us saying I'm not able to observe the data. They come to us and say, "I have a problem. My CRM and ERP system which are pretty classic, they don't align. I have my challenge where the sales team is doing all this, but I'm not able to see coming out on the other end so I can tie up those numbers." That's kind of the questions we get. How do we improve on data quality? How do we improve on process quality? Those are the kind of conversations we start with. And of course, from engineering standpoint, we are moving from a legacy into the cloud. Can you help us?
Kris Jenkins (43:51):
Yeah, yeah. Conway's Law has delivered us two systems which don't talk to each other. How can we get them talking to each other without tightly coupling them? Yeah, I can see that. Well, I think that question's going to be with us a while, but I'm glad we're all chipping away at it.
Rama Ryali (44:08):
Me too.
Kris Jenkins (44:09):
We have RightData here at the event space. Thank you very much for joining us, Rama. It's been really interesting, and I think we've invented a new buzz term with data socialization. We'll have a glossary in the show notes.
Rama Ryali (44:22):
I'm going to shamelessly start using it with your permission, Kris.
Kris Jenkins (44:25):
Please do. I get a hundredth of a cent every time you use it. You can buy me a drink the next time we meet.
Rama Ryali (44:30):
There you go. Absolutely. You know what? We'll be in London in March for the AI big data thing. So we'll probably will hook you up.
Kris Jenkins (44:38):
Excellent.
Rama Ryali (44:38):
Let's do that.
Kris Jenkins (44:39):
We'll try and bump into each other. Rama, thanks for joining us. Cheers.
Rama Ryali (44:42):
Hey, thanks Kris. You stay safe and good catching up again. Thank you again. Bye..
Kris Jenkins (44:45):
That was Rama Ryali of RightData. And I find myself wondering, what conclusions can we draw from all that? Well, one, I think is that Excel and SQL will always be with us, but they'll never be the whole of the story. No one tool will. Another is that making sure that everyone can get the maximum value out of the data in our organization is always going to be an iterative process. It's always going to be an ongoing task. There's always more value to be unlocked today and more to do tomorrow to unlock even more value in the future. And it will always be supported by tools and processes, but the sense that it's ongoing will never leave us. And the other thing that will never leave us, perhaps our biggest one, is that for a hope of having democratic access to our data, we have to engage in this social process of talking to each other about what our data is and what matters to different people and how we can gather this data and transmit it.
Kris Jenkins (45:51):
The conversation is ongoing. The need to reach across departments and across different skill sets, it can't be automated away. It can be enhanced by automation, but it will always be with us. Perhaps you could say that there's no democracy without society, but that's kind of straying into philosophy so I'm going to leave it there. And I'd love to hear your thoughts if you've got them. So if you are watching this on YouTube, please leave a comment or a like. And otherwise, you can find my Twitter handle in the show notes. I'd love to hear from you.
Kris Jenkins (46:23):
And if there's a topic that's burning in your heart and you want to come on Streaming Audio and discuss it, please do drop me a line about that, same channels. In the meantime, let me remind you that Streaming Audio is brought to you by Confluent Developer, our site that teaches you everything we know about communicating with events using Kafka. Whether you're getting started or looking to up your game, you'll find a course, a blog post, a tutorial for you. So check it out at developer.confluent.io. And with that, it remains for me to thank Rama Ryali of RightData for joining us and you for listening. I've been your host, Kris Jenkins, and I will catch you next time.